MSU Learning-based Image Compression Benchmark 2024
Explore the Best Learned Image Compression Methods
Roman Kazantsev
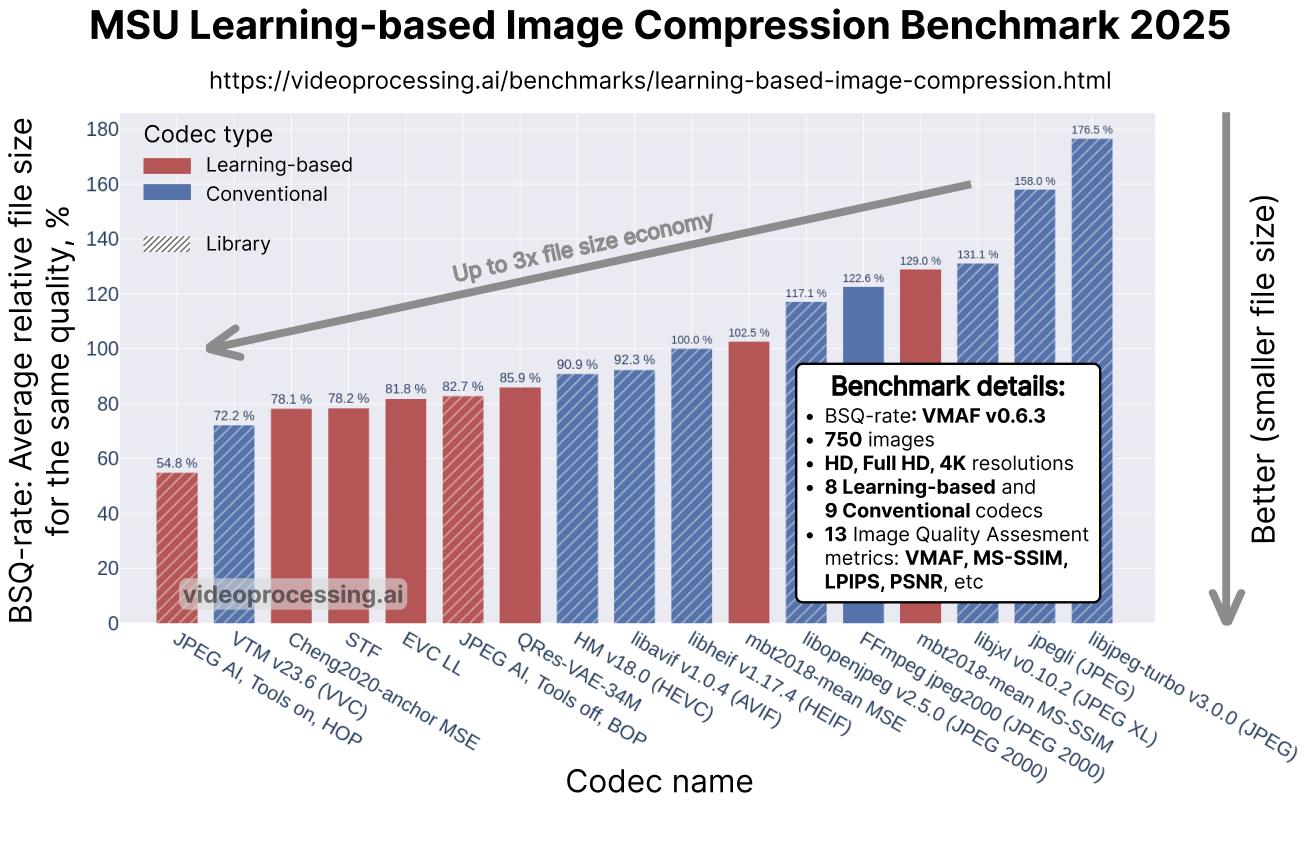
Diverse dataset
- Over 750 test images
- HD, Full HD and 4K resolutions
- Various content types
- Processed over 1M images to create the dataset
Large comparison
- 20 codecs tested
- 13 IQA metrics
- Subjective comparison (soon)
- JPEG AI reference codec results
Large leaderboard
- BSQ-rate for codec ranking
- Rate-Distortion curves
What’s new
- 14.04.2024 Benchmark Release!
- 14.02.2025 Added Jpeg AI.
Leaderboard
RD-curve examples
How to participate
Compare your codec with the best conventional and learning-based codecs. We kindly invite you to participate in our benchmark. To do this, follow the steps below:
Send us an email to image-compression-benchmark@videoprocessing.ai
with the following information:
type — encode / decode
ref — path to reference image / path to compressed file
out — path to store compressed file / path to store decompressed image
qp — quality parameter / target bpp (for encoding mode only)
|
Also you can test your codec locally on open part of Learning-Based Image Compression Benchmark dataset.
If you have any suggestions or questions, please contact us.
Cite us
@misc{rylov2024learning
title={Learning-based Image Compression Benchmark},
author={Rylov, Vitaly and Kazantsev, Roman and Vatolin, Dmitriy},
year={2024},
url={https://videoprocessing.ai/benchmarks/learning-based-image-compression.html}
}
|
Contacts
We would highly appreciate any suggestions and ideas on how to improve our benchmark.
Please contact us via e-mail: image-compression-benchmark@videoprocessing.ai.
Also, you can subscribe to updates on our benchmark:
MSU Video Quality Measurement Tool
Widest Range of Metrics & Formats
- Modern & Classical Metrics SSIM, MS-SSIM, PSNR, VMAF and 10+ more
- Non-reference analysis & video characteristics
Blurring, Blocking, Noise, Scene change detection, NIQE and more
Fastest Video Quality Measurement
- GPU support
Up to 11.7x faster calculation of metrics with GPU - Real-time measure
- Unlimited file size
Main MSU VQMT page on compression.ru
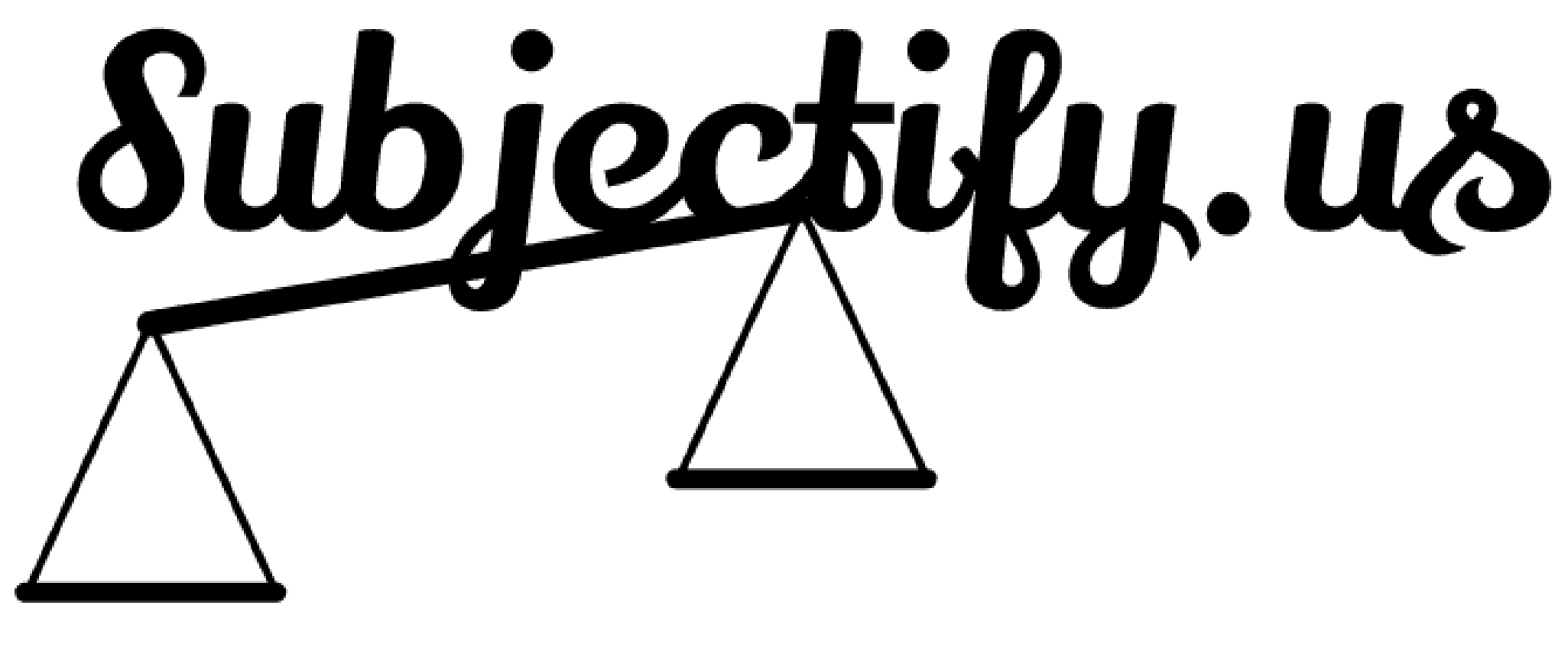
Crowd-sourced subjective
quality evaluation platform
- Conduct comparison of video codecs and/or encoding parameters
What is it?
Subjectify.us is a web platform for conducting fast crowd-sourced subjective comparisons.
The service is designed for the comparison of images, video, and sound processing methods.
Main features
- Pairwise comparison
- Detailed report
- Providing all of the raw data
- Filtering out answers from cheating respondents
Subjectify.us
-
MSU Benchmark Collection
- Learning-Based Image Compression Benchmark
- Super-Resolution for Video Compression Benchmark
- Video Colorization Benchmark
- Defenses for Image Quality Metrics Benchmark
- Super-Resolution Quality Metrics Benchmark
- Deinterlacer Benchmark
- Video Saliency Prediction Benchmark
- Metrics Robustness Benchmark
- Video Upscalers Benchmark
- Video Deblurring Benchmark
- Video Frame Interpolation Benchmark
- HDR Video Reconstruction Benchmark
- No-Reference Video Quality Metrics Benchmark
- Full-Reference Video Quality Metrics Benchmark
- Video Alignment and Retrieval Benchmark
- Mobile Video Codecs Benchmark
- Video Super-Resolution Benchmark
- Shot Boundary Detection Benchmark
- The VideoMatting Project
- Video Completion
- Codecs Comparisons & Optimization
- VQMT
- MSU Datasets Collection
- Metrics Research
- Video Quality Measurement Tool 3D
- Video Filters
- Other Projects