MSU Video Deblurring Benchmark
The most comprehensive comparison of video deblurring methods
Egor Chistov
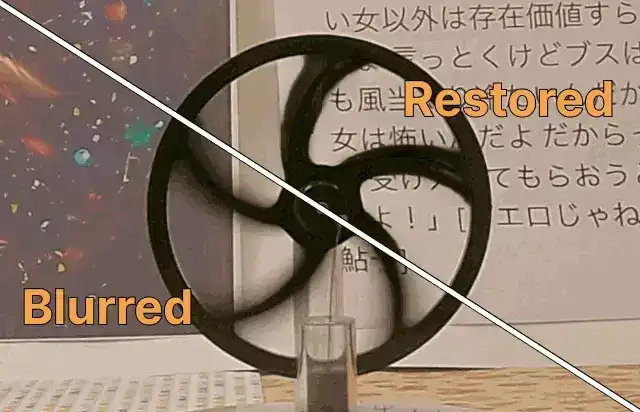
Key features of the Benchmark
- A new private real motion blur dataset for testing: 23 different scenes
- Comparison of 9 methods of video deblurring: VRT, NAFNet and more
- 5 metrics for restoration quality assessment: PSNR, SSIM, VMAF and more
- (Soon) Subjective comparison
What’s new
- 24.10.2022 Beta-version release.
Leaderboard
Click on the labels to sort the table.
In the methodology you can read brief information about all metrics.
You can scroll the table to see all the results.
Rank | Model | Y-PSNR | Y-SSIM | Y-VMAF | LPIPS | ERQAv2.0 |
---|---|---|---|---|---|---|
1 | NAFNet (REDS*) | 30.54803 | 0.95035 | 66.85941 | 0.08561 | 0.74508 |
2 | MAXIM (REDS*) | 30.65728 | 0.94959 | 67.3502 | 0.07836 | 0.74277 |
3 | Restormer | 31.76111 | 0.94632 | 66.3964 | 0.08239 | 0.74776 |
4 | VRT (REDS*) | 30.97878 | 0.94601 | 66.81782 | 0.08248 | 0.75056 |
5 | MPR local | 31.65037 | 0.94542 | 67.01788 | 0.08323 | 0.74521 |
6 | VRT (GoPro*) | 31.42945 | 0.94503 | 66.72253 | 0.08165 | 0.74874 |
7 | Deeprft (GoPro*) | 31.57612 | 0.94484 | 66.55057 | 0.08326 | 0.74323 |
8 | Deeprft (REDS*) | 31.32349 | 0.94479 | 66.46811 | 0.08139 | 0.74339 |
9 | MAXIM (GoPro*) | 31.36344 | 0.94386 | 67.7557 | 0.09188 | 0.74444 |
10 | DeblurGAN Inception | 31.17171 | 0.94301 | 66.91781 | 0.08867 | 0.74297 |
11 | Restormer local | 31.12341 | 0.94217 | 65.25911 | 0.08251 | 0.73875 |
* Train dataset
Charts
In this section you can observe the values of different metrics for each individual video and the average values. In the "Video" selector, you can observe the names of the videos. For your convenience, the methodology page has a preview of each video and its full name match.
Metric: Video:

Visualization
This section presents visualizations of all algorithms.
- The first line is full-sized frames
- The second line is crops from them
- The third and fourth lines are the visualizations of error maps of PSNR and SSIM respectively
Note that only 5 videos from dataset are publicly available now.
Video:
Model 1: Model 2: Model 3:
Drag a red rectangle in the area, which you want to crop.
GT
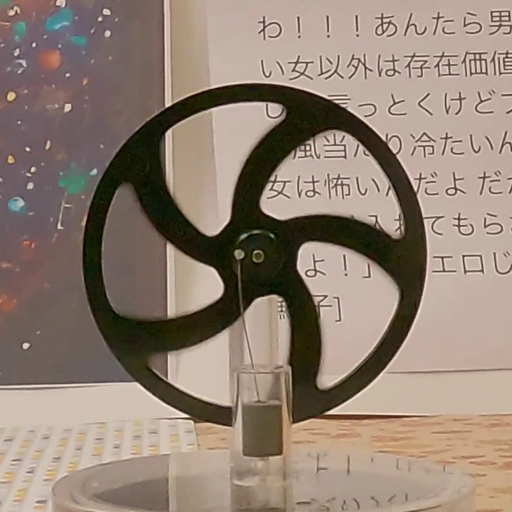
GT
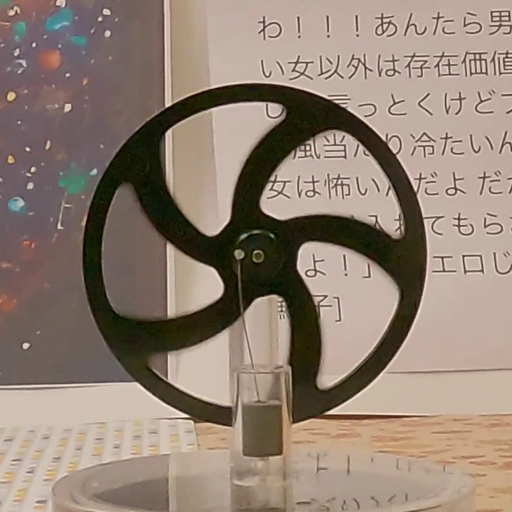
RIFE
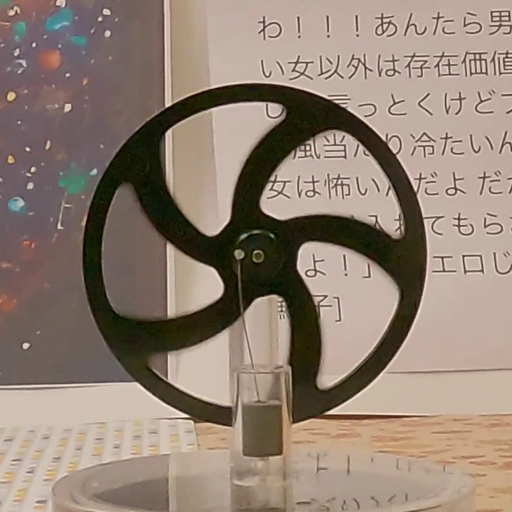
CAIN
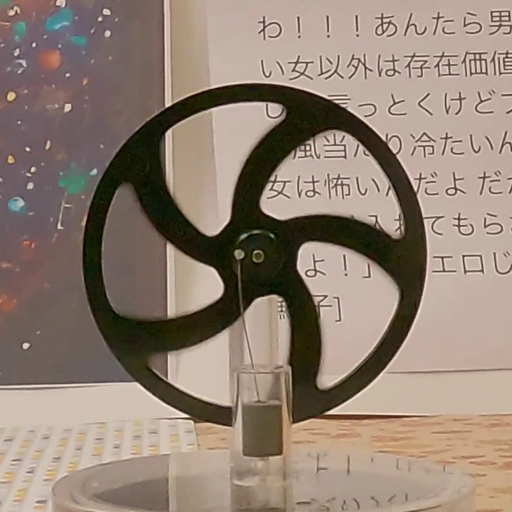
Deblurring Benchmark Roadmap
Feature | Description | Release date |
---|---|---|
Subjective comparison | We plan to conduct a subjective comparison. The subjective comparison with many video pairs may be very expensive. If you want to support our benchmark, please contact us: deblurring-benchmark@videoprocessing.ai | Q4 2022 |
More state-of-the-art deblurring methods | New deblurring methods are constantly being developed. We will add new qualitative deblurring methods to our benchmark as they appear. We also expect developers to submit their methods to us. You can submit your method here. | Q4 2022 |
Paper on benchmark/dataset | We plan to publish a paper on the presented benchmark and futhermore explain our methodology | Q1 2023 |
A new metric to measure video deblurring restoration quality | We believe that the most popular video quality metrics — PSNR and SSIM — are not applicable to the deblurring task. We are researching our metric for blurred video restoration quality that will correlate well with subjective scores. | Q2 2023 |
Submit Your Method
Verify your method’s ability to restore blurred videos and compare it with other algorithms.
You can go to the page with information about other participants.
1. Download input data
|
Download blurred videos |
|
2. Apply your algorithm |
Deblur videos using your algorithm. You can also send us the code of your method or the executable file and we will run it ourselves. |
|
3. Send us result |
Send us an email to deblurring-benchmark@videoprocessing.ai
with the following information:
|
You can verify the results of current participants or estimate the perfomance of your method on public samples of our dataset. Just send an email to deblurring-benchmark@videoprocessing.ai with a request to share them with you.
Our policy: we share only public samples of our dataset
Contact Us
For questions and propositions, please contact us: deblurring-benchmark@videoprocessing.ai
You can subscribe to updates on our benchmark:
MSU Video Quality Measurement Tool
Widest Range of Metrics & Formats
- Modern & Classical Metrics SSIM, MS-SSIM, PSNR, VMAF and 10+ more
- Non-reference analysis & video characteristics
Blurring, Blocking, Noise, Scene change detection, NIQE and more
Fastest Video Quality Measurement
- GPU support
Up to 11.7x faster calculation of metrics with GPU - Real-time measure
- Unlimited file size
Main MSU VQMT page on compression.ru
-
MSU Benchmark Collection
- Learning-Based Image Compression Benchmark
- Super-Resolution for Video Compression Benchmark
- Video Colorization Benchmark
- Defenses for Image Quality Metrics Benchmark
- Super-Resolution Quality Metrics Benchmark
- Deinterlacer Benchmark
- Video Saliency Prediction Benchmark
- Metrics Robustness Benchmark
- Video Upscalers Benchmark
- Video Deblurring Benchmark
- Video Frame Interpolation Benchmark
- HDR Video Reconstruction Benchmark
- No-Reference Video Quality Metrics Benchmark
- Full-Reference Video Quality Metrics Benchmark
- Video Alignment and Retrieval Benchmark
- Mobile Video Codecs Benchmark
- Video Super-Resolution Benchmark
- Shot Boundary Detection Benchmark
- The VideoMatting Project
- Video Completion
- Codecs Comparisons & Optimization
- VQMT
- MSU Datasets Collection
- Metrics Research
- Video Quality Measurement Tool 3D
- Video Filters
- Other Projects