MSU Video Super-Resolution Benchmark: Detail Restoration — find the best upscaler
Discover the newest methods and find the most appropriate method for your tasks
Eugene Lyapustin
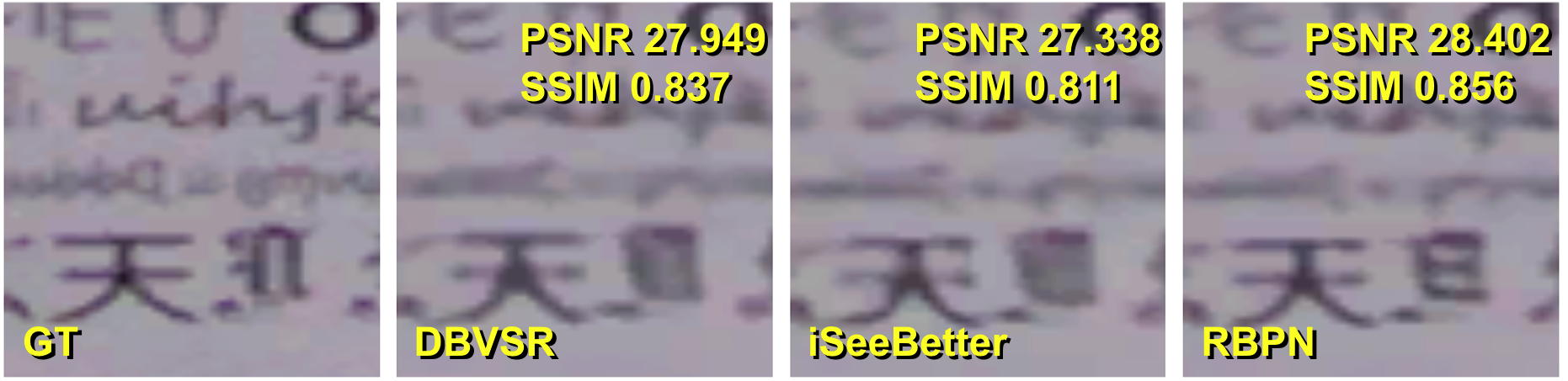
What’s new
- 12.06.2023 Added 6 new algorithms
- 06.04.2022 Added 8 new algorithms and LPIPS metric
- 15.11.2021 Our paper "ERQA: Edge-restoration Quality Assessment for Video Super-Resolution" was accepted to VISAPP 2022
- 10.09.2021 Our ERQA metric was published on GitHub and can be installed with pip install erqa
Click here to see more news
- 26.08.2021 Added 8 new algorithms
- 15.07.2021 Improved Visualization section to be more user-friendly and added plots with the metric difference on BI and BD degradation to Leaderboard section
- 26.04.2021 Beta-version Release
Key features of the Benchmark
- New metrics for the detail restoration quality
- Check methods’ ability to restore real details
- The most complex content for restoration task: faces, text, QR-codes, car numbers, unpatterned textures, small details
- See plots and visualizations for particular content types
- Different degradation types to lower the resolution: bicubic interpolation (BI) and Gaussian blurring and downsampling (BD), with and without noise
- Many methods use only one degradation type for their training datasets (e.g. bicubic interpolation) and do not work well on others. Choose the method that didn’t overfit the test dataset
- Subjective comparison of new and popular Super-Resolution methods
Leaderboard
The table below shows a comparison of Video Super Resolution methods by subjective score and a few objective metrics. Default ranking is by subjective score. You can click on the model's name in the table to read information about the method. You can see information about all participants here.
Rank | Model | Subjective | ERQAv1.0 | LPIPS | SSIM-Y** | QRCRv1.0 | PSNR-Y** |
---|
Charts
In this section, you can see barcharts and speed-to-performance plots. You can choose metric, motion, content, and degradation type, see tests with or without noise.
You can see information about all participants here.
Metric: Test: Content:
Metric: Test: Content:
Highlight the plot region where you want to zoom in
Visualization
In this section, you can choose a part of the frame with particular content, see a cropped piece from this, MSU VQMT PSNR* Visualization, and ERQAv1.0 Visualization for this crop.
In part "QR-codes" codes, which can be detected, are surrounded by a blue rectangle. You can see information about all participants here.
*We visualize shifted PSNR metric by applying MSU VQMT PSNR Visualization to frames with optimal shift for PSNR.
Frame: Content:
Model 1: Model 2: Model 3:
Drag a red rectangle in the area, which you want to crop.
GT
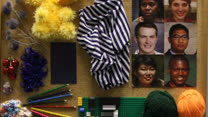
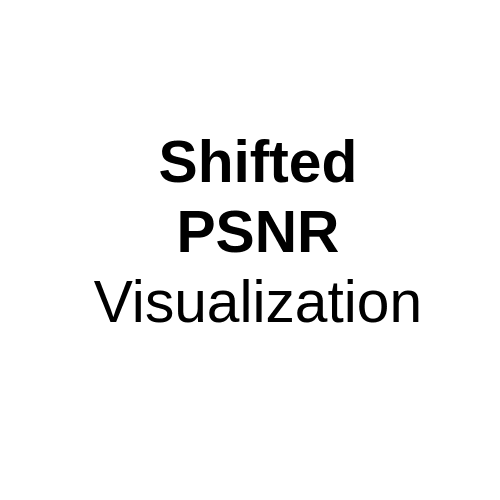
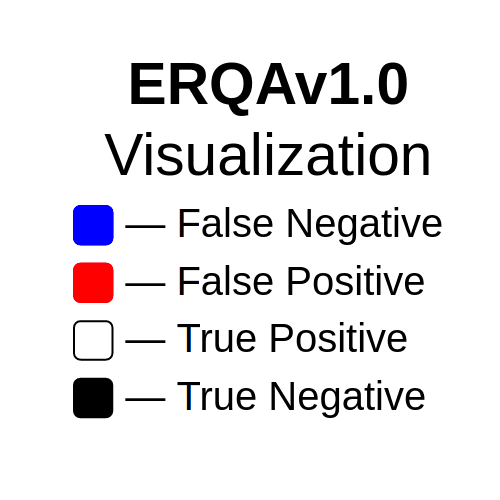
D3Dnet
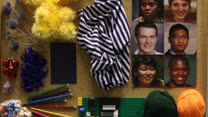
DBVSR
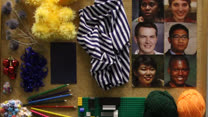
DUF-16L
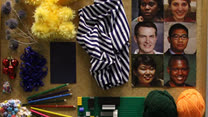
Your method submission
Verify the restoration ability of your VSR algorithm and compare it with state-of-the-art solutions.
You can see information about all other participants here.
1. Download input data
|
Download input low-resolution videos as sequences of frames in .png format
There are 2 available options:
Neighboring videos are separated by 10 black frames, which will be skipped for evaluation.
with 100 frames each here. |
|
|
||
2. Apply your algorithm |
Restore high-resolution frames with your algorithm. You can also send us the code of your method or the executable file and we will run it ourselves. |
|
|
||
3. Send us result |
Send us an email to vsr-benchmark@videoprocessing.ai
with the following information:
Check that the number of output frames matches the number of input frames:
|
Contact Us
For questions and propositions, please contact us: vsr-benchmark@videoprocessing.ai
Cite Us
To refer to our benchmark or metric ERQA in your work, cite one of our papers:
@conference{
author={Anastasia Kirillova and Eugene Lyapustin and Anastasia Antsiferova and Dmitry Vatolin},
title={ERQA: Edge-restoration Quality Assessment for Video Super-Resolution},
booktitle={Proceedings of the 17th International Joint Conference on Computer Vision, Imaging and Computer Graphics Theory and Applications - Volume 4: VISAPP,},
year={2022},
pages={315-322},
publisher={SciTePress},
organization={INSTICC},
doi={10.5220/0010780900003124},
isbn={978-989-758-555-5},
}
|
MSU Video Quality Measurement Tool
Widest Range of Metrics & Formats
- Modern & Classical Metrics SSIM, MS-SSIM, PSNR, VMAF and 10+ more
- Non-reference analysis & video characteristics
Blurring, Blocking, Noise, Scene change detection, NIQE and more
Fastest Video Quality Measurement
- GPU support
Up to 11.7x faster calculation of metrics with GPU - Real-time measure
- Unlimited file size
Main MSU VQMT page on compression.ru
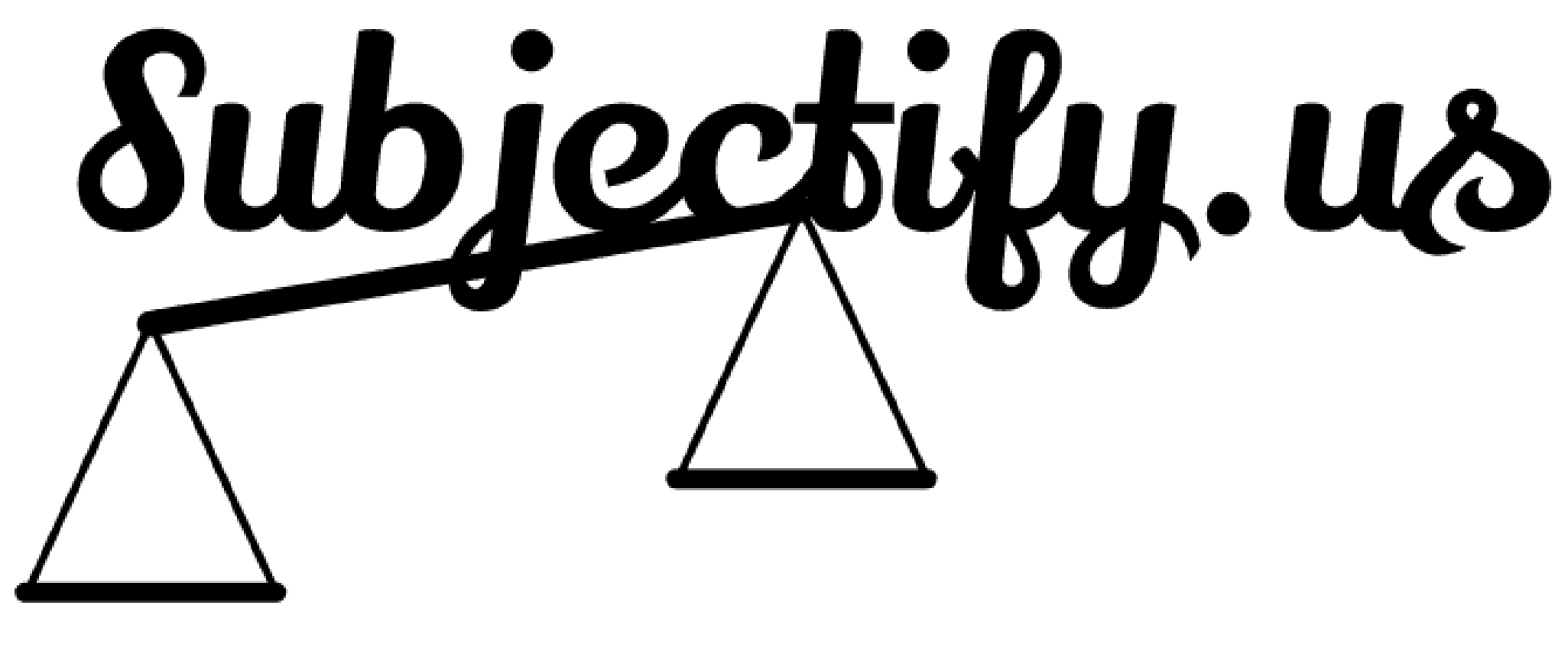
Crowd-sourced subjective
quality evaluation platform
- Conduct comparison of video codecs and/or encoding parameters
What is it?
Subjectify.us is a web platform for conducting fast crowd-sourced subjective comparisons.
The service is designed for the comparison of images, video, and sound processing methods.
Main features
- Pairwise comparison
- Detailed report
- Providing all of the raw data
- Filtering out answers from cheating respondents
Subjectify.us
-
MSU Benchmark Collection
- Learning-Based Image Compression Benchmark
- Super-Resolution for Video Compression Benchmark
- Video Colorization Benchmark
- Defenses for Image Quality Metrics Benchmark
- Super-Resolution Quality Metrics Benchmark
- Deinterlacer Benchmark
- Video Saliency Prediction Benchmark
- Metrics Robustness Benchmark
- Video Upscalers Benchmark
- Video Deblurring Benchmark
- Video Frame Interpolation Benchmark
- HDR Video Reconstruction Benchmark
- No-Reference Video Quality Metrics Benchmark
- Full-Reference Video Quality Metrics Benchmark
- Video Alignment and Retrieval Benchmark
- Mobile Video Codecs Benchmark
- Video Super-Resolution Benchmark
- Shot Boundary Detection Benchmark
- The VideoMatting Project
- Video Completion
- Codecs Comparisons & Optimization
- VQMT
- MSU Datasets Collection
- Metrics Research
- Video Quality Measurement Tool 3D
- Video Filters
- Other Projects