Real-World Stereo Color and Sharpness Mismatch Dataset
How to film stereoscopic dataset with real color and sharpness mismatches?
Key Features
- 14 videos: 7 stereopairs each with 4K cropped to 960x720 resolution
- Only real-world stereoscopic color and sharpness mismatch distortions
- Check how your method performs on real-world data
Download
You can download our dataset by clicking this button.
Dataset Preview
In this section you can observe typical left distorted and left ground truth views from our dataset. Note that these views have different brightness and sharpness characteristics. Right view shares the same characteristics with the left ground truth view. Regions of interest are highlighted with white rectangles.
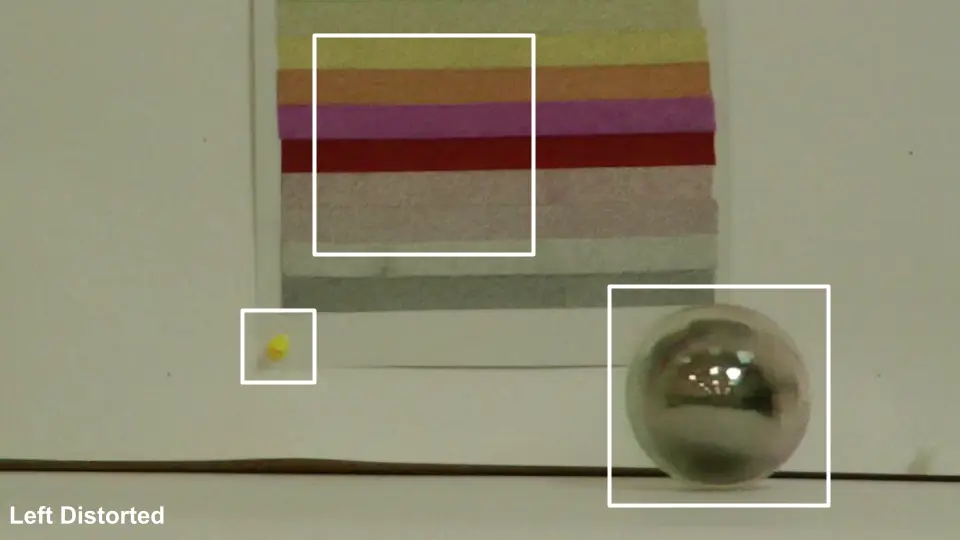
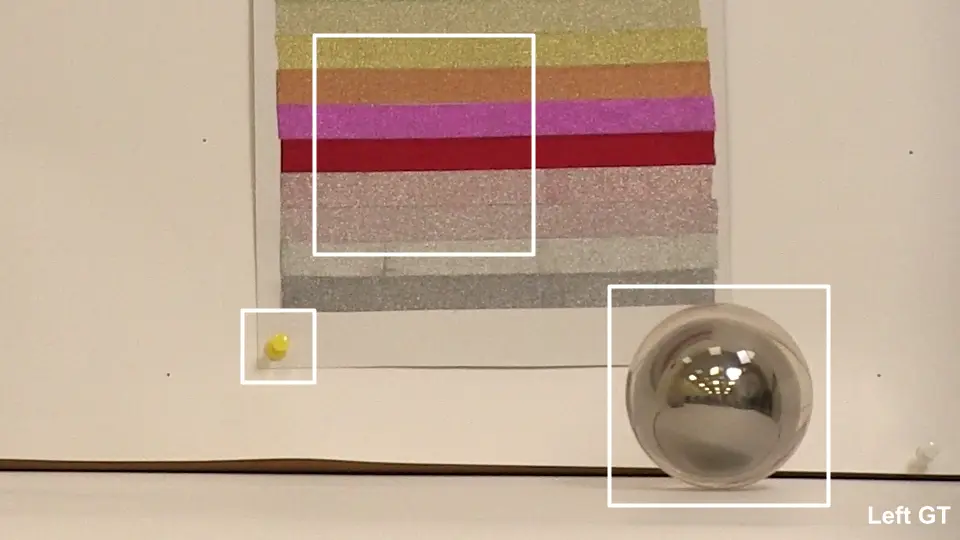
Drag the separator to see differences between two views
Dataset Scenes
In this section you can observe typical scenes from our dataset. Our dataset contains moving objects and light sources. We prefer objects that have complex textures or produce glares to ensure dataset complexity.
Press the play button to watch selected scene
Methodology
Color and sharpness mismatches between views of the stereoscopic 3D video can decrease the overall video quality and may cause viewer discomfort and headaches. To eliminate this problem, there are correction methods that aim to make the views consistent.
We propose a new real-world dataset of stereoscopic videos for evaluating color-mismatch-correction methods. We collected it using a beam splitter and three cameras. A beam splitter introduces real-world mismatches between stereopair views. Similar mismatches can appear in stereoscopic movies filmed with a beam-splitter rig. Our approach used a beam splitter to set a zero stereobase between the left camera and the left ground-truth camera, allowing us to create a distorted ground-truth data pair.
Filming the left distorted view and left ground-truth view without parallax is crucial to achieving precise ground-truth data. Postprocessing can precisely correct affine mismatches between views—namely scale, rotation angle, and translation—but it cannot correct parallax without using complex optical-flow, occlusion-estimation, and inpainting algorithms, which can reduce ground-truth-data quality. We achieved zero parallax through our method, but the manual alignment was time consuming.
Dataset filming and postprocessing pipeline
Our research employed the following postprocessing pipeline. Given the three videos from our cameras, we matched the horizontally flipped left distorted view to the left ground-truth view and rectified the stereopair using a homography transformation. To estimate the transformation parameters, we employed MAGSAC++. We selected SIFT and the Brute-Force matcher to match the left views, as well as the LoFTR matcher for rectification because it can better handle large parallax.
The monge-kantorovitch linear colour mapping perfectly corrected the small loss in the transmitted beam. Without it, our approach would have been unable to obtain accurate ground-truth data. We performed temporal alignment using audio streams captured by the cameras: superimposing one stream on the other in the video editor allowed us to find the offset in frames.
Postprocessing scripts are at this repo.
Other Datasets
In this section you can observe key parameters of multiple datasets for color-mismatch-correction task. Previous datasets for this task either are too small or lack real-world mismatch examples.
Dataset | Source Data Size | Applied Distortions |
---|---|---|
Niu et al. [1] | 18 frame pairs from 2D videos captured within a short period (no longer than 2 seconds) that share the same color feature | Photoshop CS6 operators: saturation, brightness, contrast, hue, color balance, and exposure, each with three severity levels |
Lavrushkin et al. [2] | 1000 stereopairs without color mismatches from S3D movies produced only via 3D rendering | Simplex noise smoothed using domain transform filter |
Grogan et al. [3] | 15 image pairs from the dataset provided by Hwang et al. [4] Note that this is not a stereo image pairs dataset | Different illumination conditions, camera settings, and color touch-up styles |
Croci et al. [5] | 1035 stereo image pairs from Flick1024, InStereo2K, and IVY LAB Stereoscopic 3D image database | Photoshop 2021 operators: brightness, color balance, contrast, exposure, hue, and saturation, each with six severity levels |
Ours | 14 stereo videos filmed using beam splitter and three cameras | Beam splitter distortions including different illuminations, and different camera settings |
Citation
Please contact us by email stereo-mismatch-dataset@videoprocessing.ai if you have any questions.
@article{chistov2023color,
title={Color Mismatches in Stereoscopic Video: Real-World Dataset and Deep Correction Method},
author={Chistov, Egor and Alutis, Nikita and Velikanov, Maxim and Vatolin, Dmitriy},
howpublished={arXiv preprint arXiv:2303.06657},
year={2023}
}
References
- Y. Niu, H. Zhang, W. Guo, and R. Ji, “Image Quality Assessment for Color Correction based on Color Contrast Similarity and Color Value Difference,” in IEEE Transactions on Circuits and Systems for Video Technology, 2016, pp. 849-862.
- S. Lavrushkin, V. Lyudvichenko, and D. Vatolin, “Local Method of Color-Difference Correction between Stereoscopic-Video Views,” in 2018-3DTV-Conference: The True Vision-Capture, Transmission and Display of 3D Video (3DTV-CON), 2018 pp. 1-4.
- M. Grogan, and R. Dahyot, “L2 Divergence for Robust Colour Transfer,” in Computer Vision and Image Understanding, 2019, pp. 39-49.
- Y. Hwang, J. Y. Lee, I. So Kweon, and S. Joo Kim, “Color Transfer using Probabilistic Moving Least Squares,” in Proceedings of the IEEE Conference on Computer Vision and Pattern Recognition, 2014, pp. 3342-3349.
- S. Croci, C. Ozcinar, E. Zerman, R. Dudek, S. Knorr, and A. Smolic, “Deep Color Mismatch Correction in Stereoscopic 3D Images,” in 2021 IEEE International Conference on Image Processing (ICIP), 2021, pp. 1749-1753.
-
MSU Benchmark Collection
- Learning-Based Image Compression Benchmark
- Super-Resolution for Video Compression Benchmark
- Video Colorization Benchmark
- Defenses for Image Quality Metrics Benchmark
- Super-Resolution Quality Metrics Benchmark
- Deinterlacer Benchmark
- Video Saliency Prediction Benchmark
- Metrics Robustness Benchmark
- Video Upscalers Benchmark
- Video Deblurring Benchmark
- Video Frame Interpolation Benchmark
- HDR Video Reconstruction Benchmark
- No-Reference Video Quality Metrics Benchmark
- Full-Reference Video Quality Metrics Benchmark
- Video Alignment and Retrieval Benchmark
- Mobile Video Codecs Benchmark
- Video Super-Resolution Benchmark
- Shot Boundary Detection Benchmark
- The VideoMatting Project
- Video Completion
- Codecs Comparisons & Optimization
- VQMT
- MSU Datasets Collection
- Metrics Research
- Video Quality Measurement Tool 3D
- Video Filters
- Other Projects