List of participants of MSU Video Super-Resolution Benchmark: Detail Restoration
Name | Multi-frame | Integrate temporal information by | Training dataset | Framework | Year |
---|---|---|---|---|---|
D3Dnet | Yes | Deformable convolution | Vimeo-90k | PyTorch | 2020 |
DBVSR | Yes | Optical flow | REDS | PyTorch | 2020 |
DUF | Yes | Deformable convolution | Unpublished | TensorFlow | 2018 |
DynaVSR-V | Yes | Vimeo-90k | PyTorch | 2020 | |
DynaVSR-R | Yes | REDS | PyTorch | 2020 | |
ESPCN | No | — | ImageNet | PyTorch | 2016 |
ESRGAN | No | — | PyTorch | 2018 | |
iSeeBetter | Yes | Recurrent architecture + optical flow | PyTorch | 2020 | |
LGFN | Yes | Deformable convolution | Vimeo-90k | PyTorch | 2020 |
RBPN | Yes | Recurrent architecture + optical flow | Vimeo-90k | PyTorch | 2019 |
RRN | Yes | Recurrent architecture | Vimeo-90k | PyTorch | 2020 |
Real-ESRGAN | No | — | PyTorch | 2021 | |
Real-ESRNet | No | — | PyTorch | 2021 | |
RealSR | No | — | DF2K, DPED | PyTorch | 2020 |
RSDN | Yes | Recurrent | Vimeo-90k | PyTorch | 2020 |
SOF-VSR | Yes | Optical flow | CDVL | PyTorch | 2020 |
TDAN | Yes | Deformable convolution | Vimeo-90k | PyTorch | 2020 |
TGA | Yes | Temporal Group Attention | Vimeo-90k | PyTorch | 2020 |
TMNet | Yes | Deformable convolution | Vimeo-90k | PyTorch | 2021 |
D3Dnet
- Use a deformable 3D convolution to compensate the motion between frames implicitly.
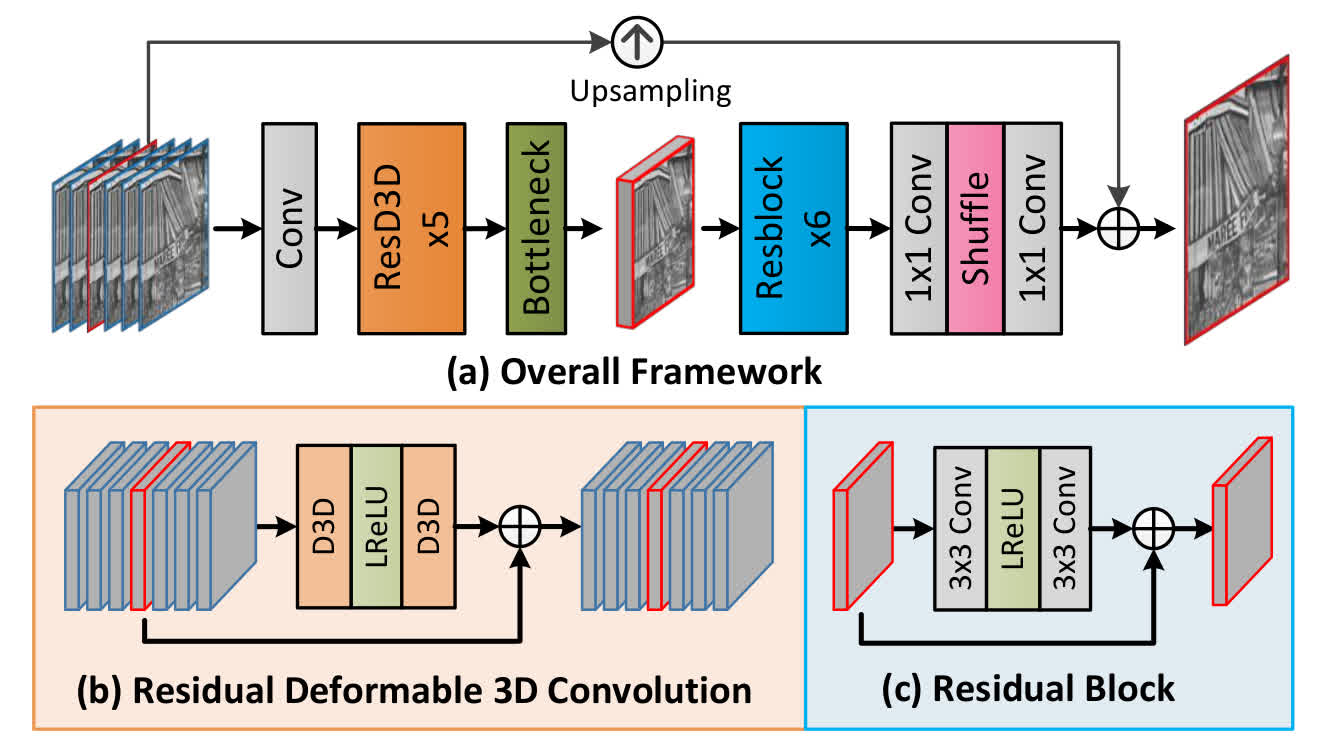
- Added to the benchmark by MSU
DBVSR
- Estimate a motion blur for the particular input. Compensate the motion between frames explicitly.
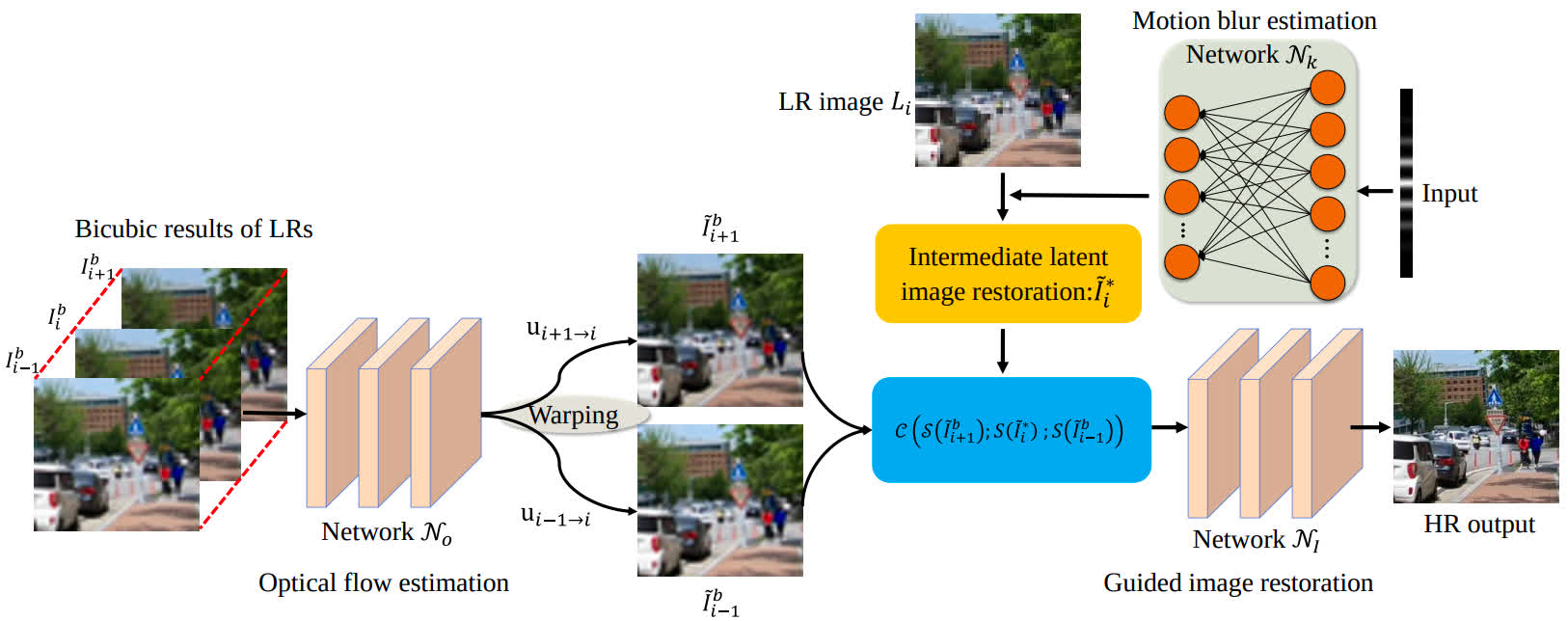
- Added to the benchmark by MSU
DUF
- Two models: DUF-16L (16 layers), DUF-28L (28 layers)
- Use a deformable 3D convolution to compensate the motion between frames implicitly.
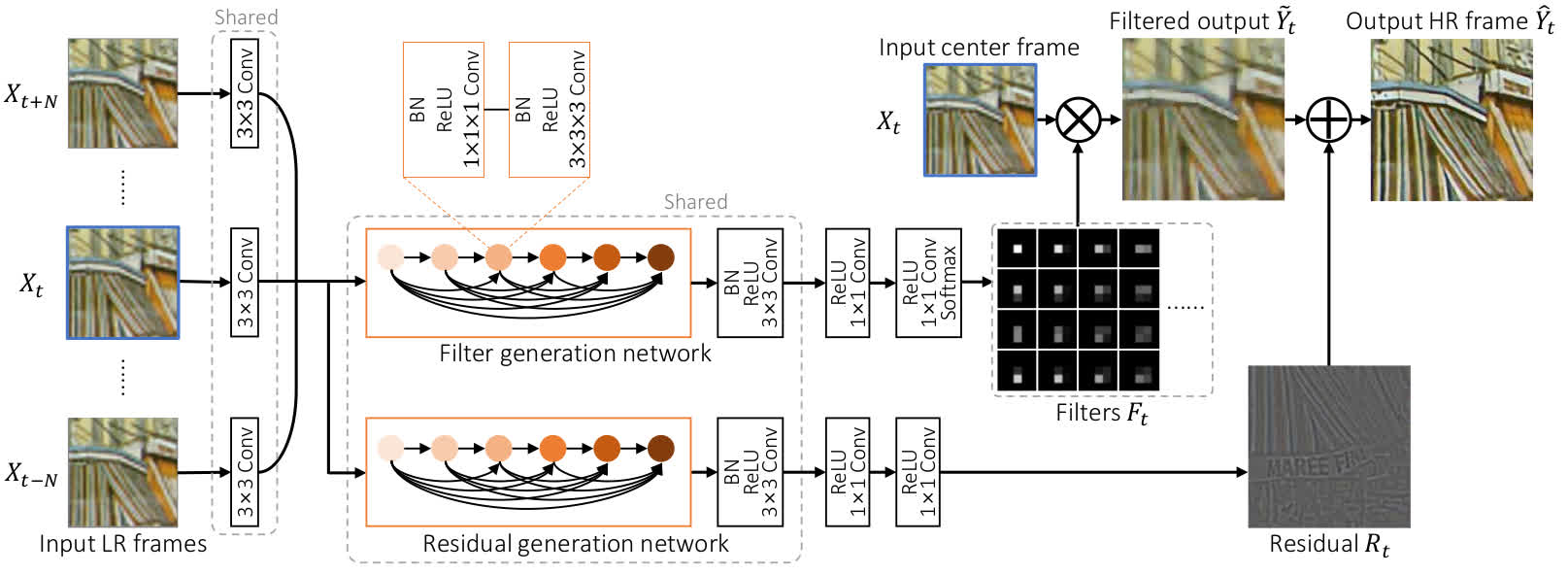
- Added to the benchmark by MSU
DynaVSR
- Use meta-learning to estimate a degradation kernel for the particular input.
- DynaVSR can be applied to any VSR deep-learning model. For our benchmark, we used pretrained weights for model EDVR, which use Deformable convolution to align neighboring frames.
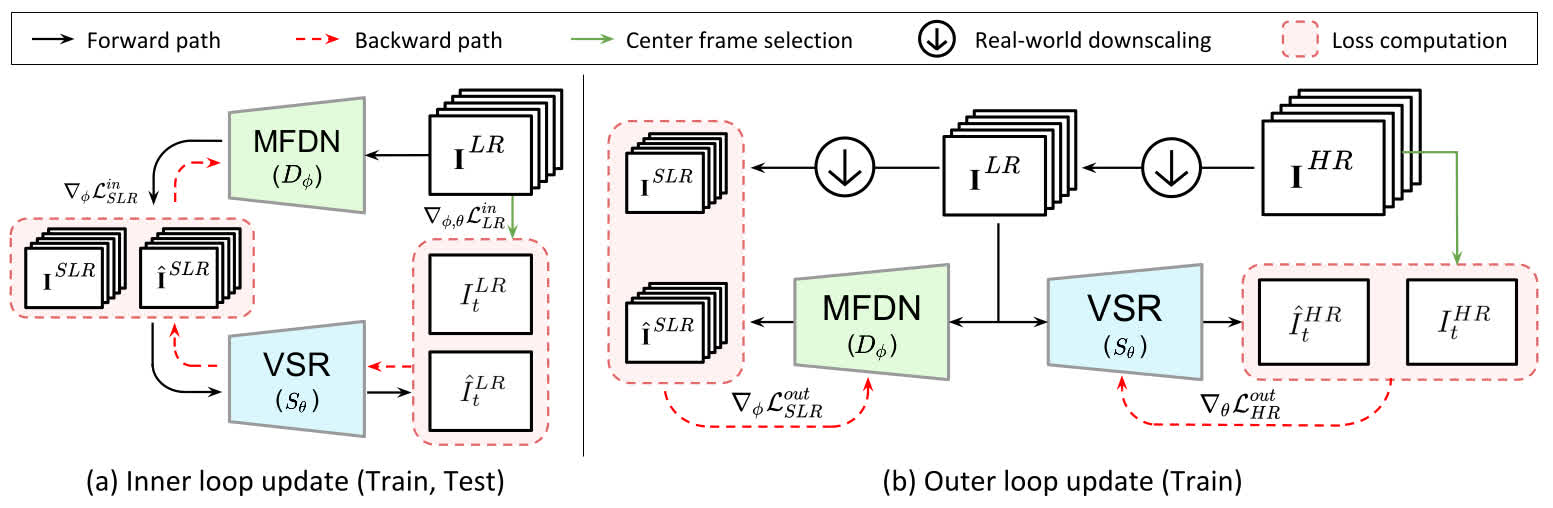
- Added to the benchmark by MSU
ESPCN
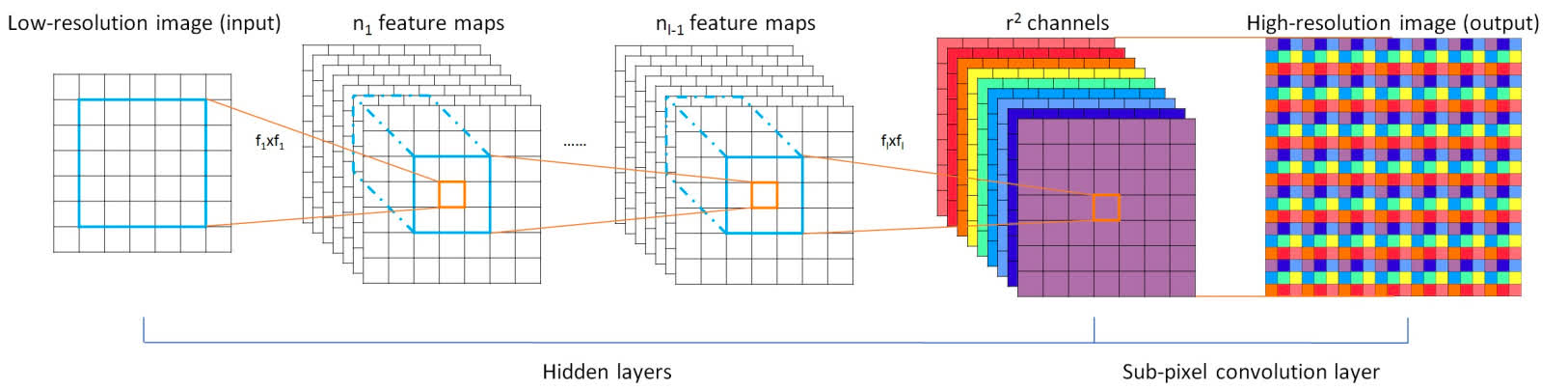
- Added to the benchmark by MSU
ESRGAN

- Added to the benchmark by MSU
iSeeBetter
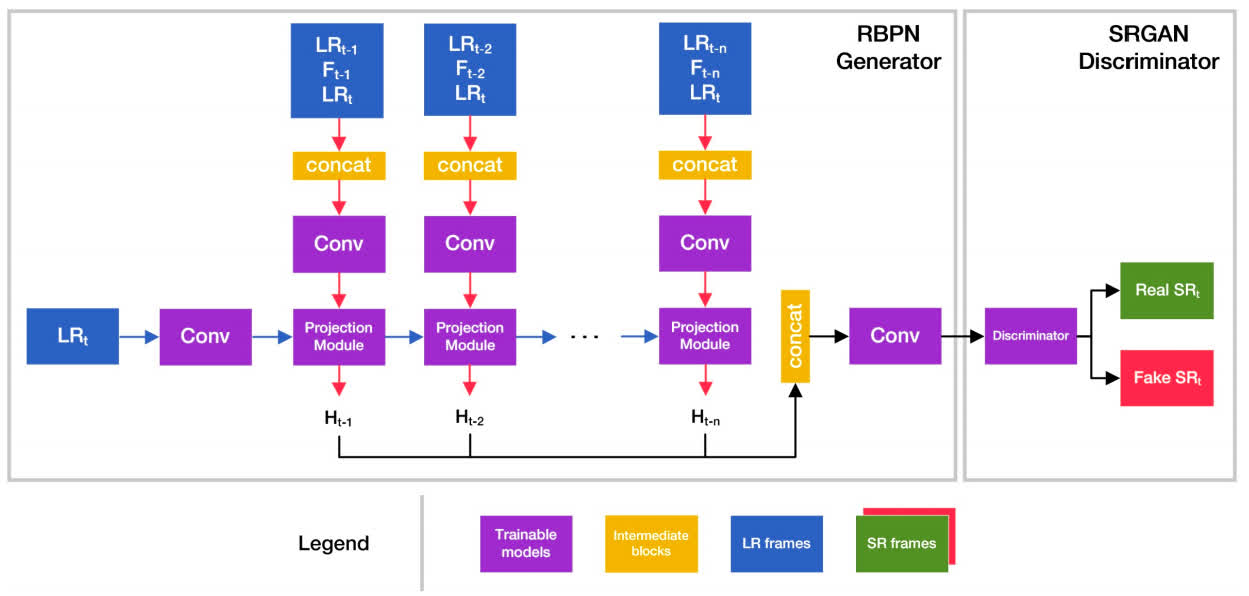
- Added to the benchmark by MSU
LGFN
- Use deformable convolutions with decreased multi-dilation convolution units (DMDCUs) to align frames explicitly. Fuse features from local and global fusion modules.
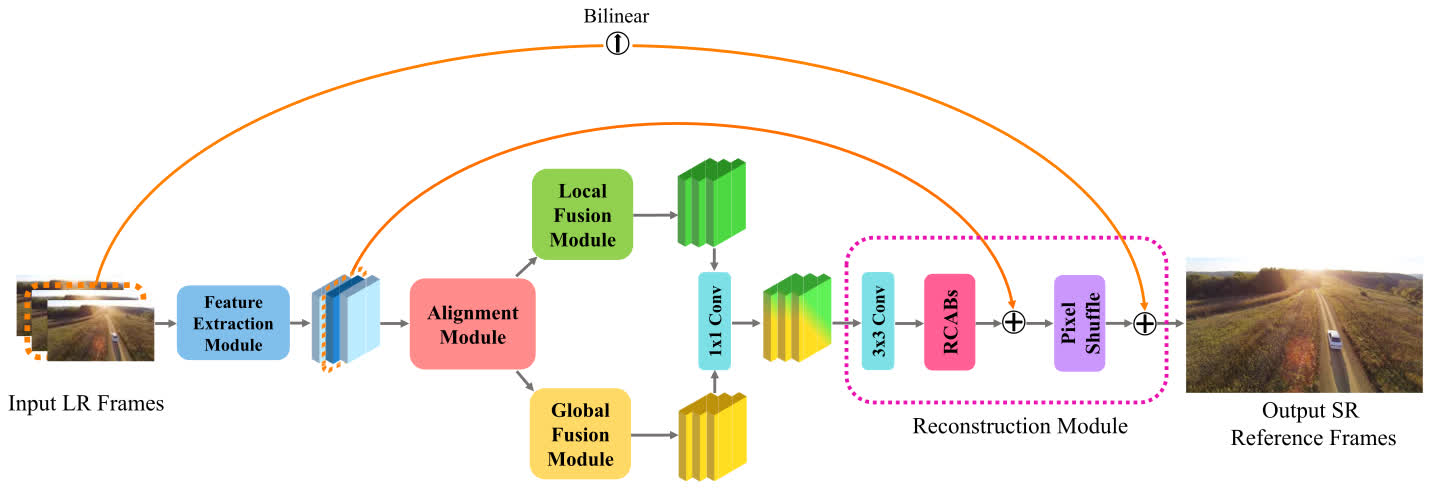
- Added to the benchmark by MSU
RBPN
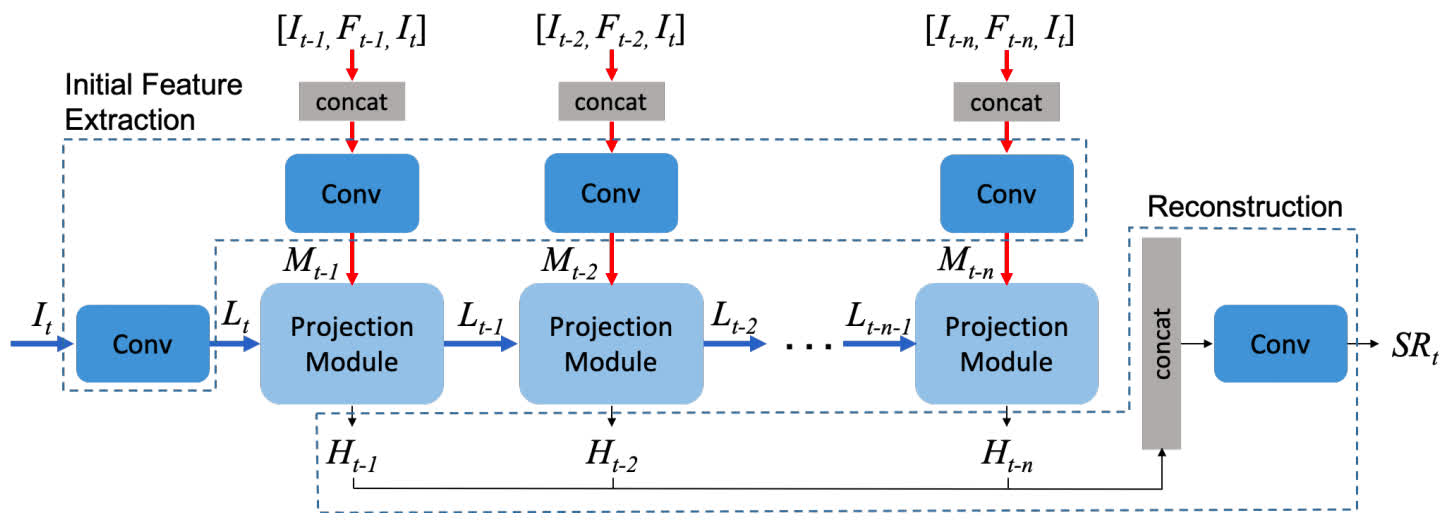
- Added to the benchmark by MSU
Real-ESRGAN
- Added to the benchmark by MSU
Real-ESRNet
- Added to the benchmark by MSU
RealSR
- Try to estimate degradation kernel and noise distribution for better visual quality.
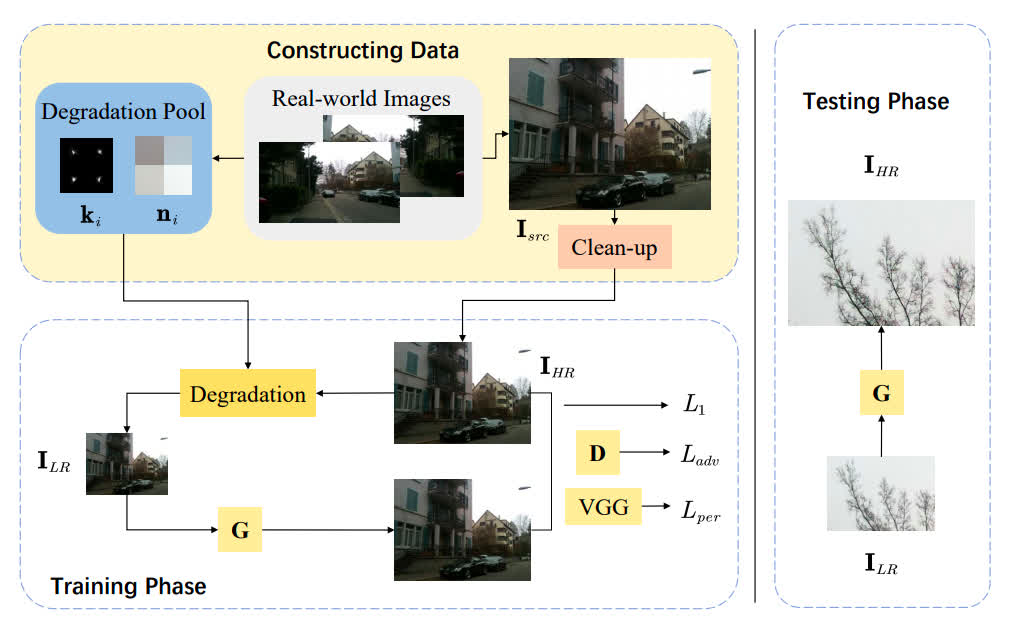
- Added to the benchmark by MSU
RRN
- Two models: RRN-5L (five residual blocks), RRN-10L (ten residual blocks)
- Use recurrent strategy with sets of residual blocks to store information from previous frames.
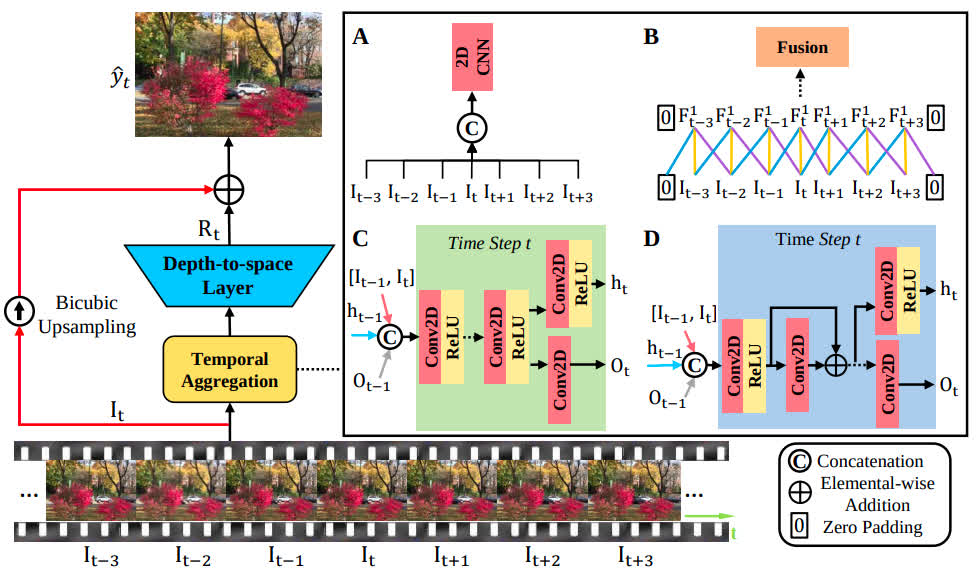
- Added to the benchmark by MSU
RSDN
- It divides the input into structure and detail components which are fed to a recurrent unit composed of several proposed two-stream structure-detail blocks.
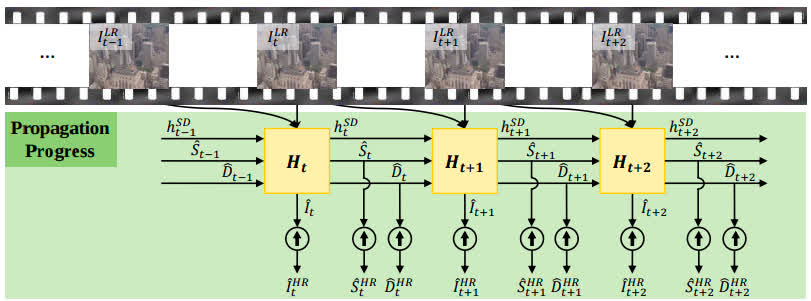
- Added to the benchmark by MSU
SOF-VSR
- Two models: SOF-VSR-BD (trained on gauss degradation type), SOF-VSR-BI (trained on bicubic degradation type)
- Compensate motion by high-resolution optical flow, estimated from the low-resolution one in a coarse-to-fine manner.
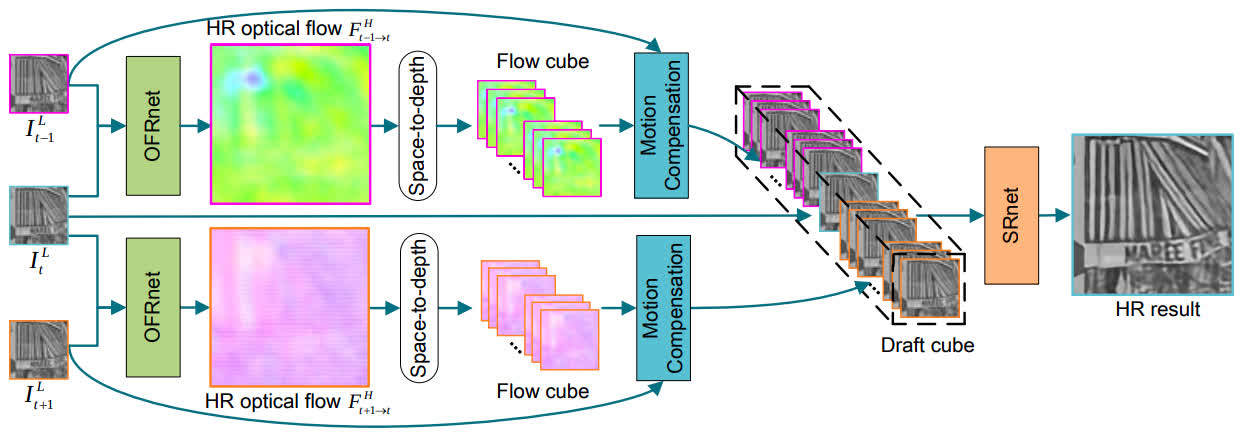
- Added to the benchmark by MSU
TDAN
- Use a deformable 3D convolution to compensate the motion between frames implicitly.

- Added to the benchmark by MSU
TGA
- The input sequence is reorganized into several groups of subsequences with different frame rates. The grouping allows to extract spatio-temporal information in a hierarchical manner, which is followed by an intra-group fusion module and inter-group fusion module.
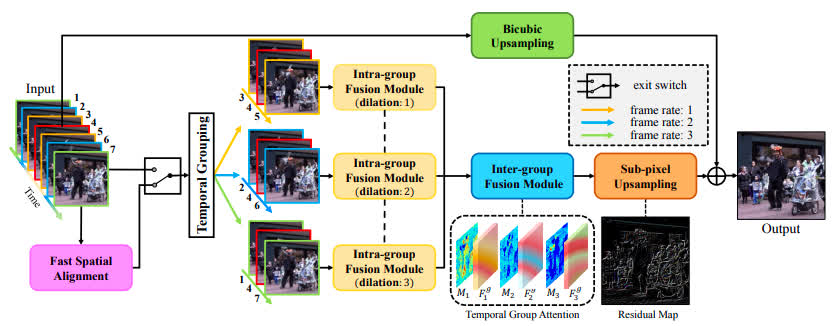
- Added to the benchmark by MSU
TMNet
- Temporal Modulation Network was trained for Space-Time Video Super Resolution. The temporal information is integrated by deformable convolution with the multi-frame input.
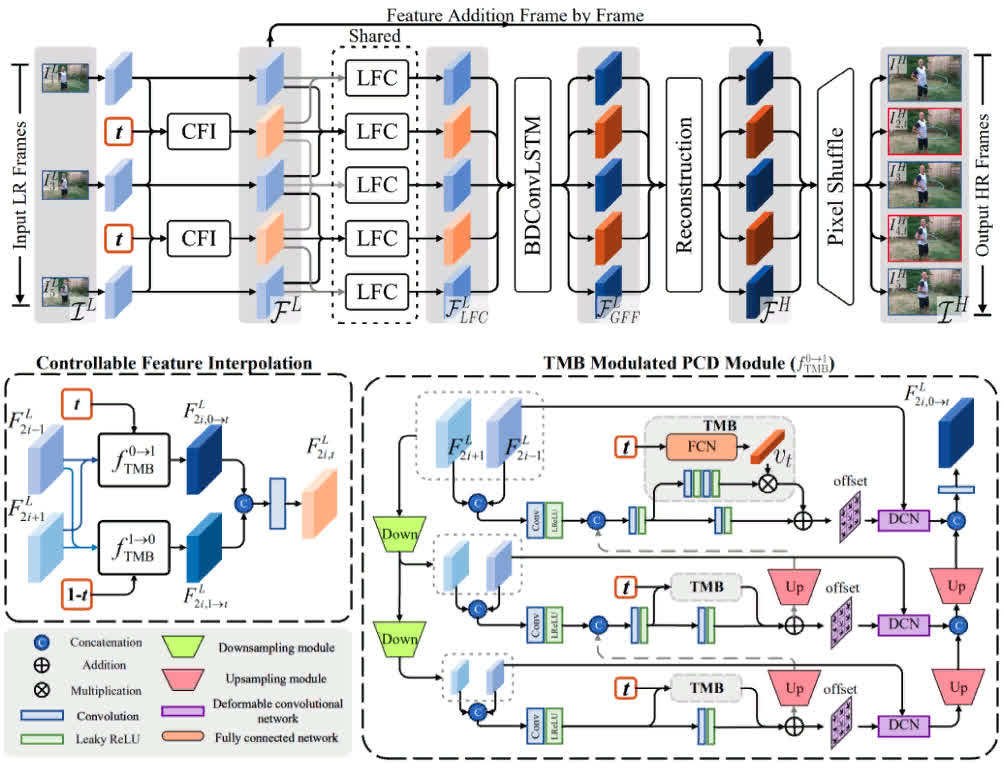
- Added to the benchmark by the author, Gang Xu.
See Also
Video Colorization Benchmark
Explore the best video colorization algorithms
Super-Resolution for Video Compression Benchmark
Learn about the best SR methods for compressed videos and choose the best model to use with your codec
Defenses for Image Quality Metrics Benchmark
Explore defenses from adv attacks
Learning-Based Image Compression Benchmark
The First extensive comparison of Learned Image Compression algorithms
Super-Resolution Quality Metrics Benchmark
Discover 66 Super-Resolution Quality Metrics and choose the most appropriate for your videos
Video Saliency Prediction Benchmark
Explore the best video saliency prediction (VSP) algorithms
Site structure
-
MSU Benchmark Collection
- Video Colorization Benchmark
- Super-Resolution for Video Compression Benchmark
- Defenses for Image Quality Metrics Benchmark
- Learning-Based Image Compression Benchmark
- Super-Resolution Quality Metrics Benchmark
- Video Saliency Prediction Benchmark
- Metrics Robustness Benchmark
- Video Upscalers Benchmark
- Video Deblurring Benchmark
- Video Frame Interpolation Benchmark
- HDR Video Reconstruction Benchmark
- No-Reference Video Quality Metrics Benchmark
- Full-Reference Video Quality Metrics Benchmark
- Video Alignment and Retrieval Benchmark
- Mobile Video Codecs Benchmark
- Video Super-Resolution Benchmark
- Shot Boundary Detection Benchmark
- The VideoMatting Project
- Video Completion
- Codecs Comparisons & Optimization
- VQMT
- MSU Datasets Collection
- Metrics Research
- Video Quality Measurement Tool 3D
- Video Filters
- Other Projects